The past years have witnessed a great deal of activity in the field of distribution testing— so much, in fact, that it has become a bit of a challenge to even keep track of what’s happening and what has already happened. While this blog post is not going to be a comprehensive (comprehensible, maybe?) survey or summary, it hopefully will at least shed some light on what distribution testing is — and where to look at if interested in it.
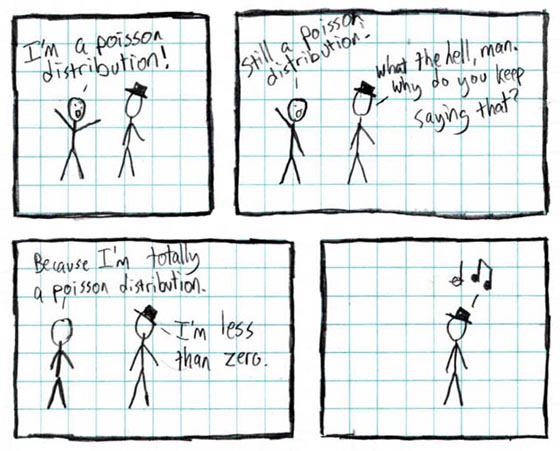
Obligatory XKCD comic.
Recall that in the “vanilla” property testing setting, one is provided with access (typically query access) to an object \(\mathcal{O}\) from some universe \(\Omega\) (typically the space of Boolean functions over \(\{0,1\}^n\) or the set of \(n\)-vertex graphs), a distance measure \(\textrm{d}(\cdot,\cdot)\) between such objects (usually the Hamming distance), a property \(\mathcal{P}\subseteq \Omega\) (the subset of “good objects”) and a parameter \(\varepsilon \in (0,1]\). The goal is to design a randomized algorithm that (i) accepts with high probability if \(\mathcal{O}\in\mathcal{P}\); and (ii) rejects with high probability if \(d(\mathcal{O},\mathcal{O}’)>\varepsilon\) for every single object \(\mathcal{O}’\in\mathcal{P}\).
The distribution testing setting is very much like this one, with of course crucial twists. The object is now a probability distribution \(D\) over a (discrete) set, typically \([n]=\{1,\dots,n\}\); the access model is usually a sample oracle, providing independent samples from \(D\) on demand; the distance is the total variation distance between distributions (or, equivalently, the \(\ell_1\) norm between the probability mass functions).
\(\textrm{d}_{\rm TV}(D,D’) = \sup_{S\subseteq [n]} \left( D(S) – D'(S) \right) = \frac{1}{2} \sum_{i\in[n]} \left|D(i)-D'(i) \right|\)
This brings in some quite important distinctions: for instance, randomness is now inherent: even taking a number of samples beyond any reasonable bound will not bring the probability of failure of the algorithm to zero, this time.
A Brief History
The field made its debut in TCS with a work of Goldreich and Ron [5], who considered the question of testing if an expander graph had mixed — that is, if the distribution induced on its nodes was uniform. However, it is only a tad later, in a work of Batu, Fortnow, Rubinfeld, Smith, and White [6] that the setting was formally defined and studied, for the specific problem of testing whether two unknown random variables have the same distribution (closeness, or equivalence testing).
This started a line of work, which analyzed the sample complexity of testing uniformity (is my random variable uniformly distributed?), identity (is it distributed according to this nice distribution I fully know in advance?), independence (are my random variables independent of each other?), monotonicity (is the probability density function increasing?), and many more. Over the course of the past 16 years, many breakthroughs, new techniques, new questions, and new answers to old problems were made and found. With surprising twists (if your domain size is n, then surely \(\Theta(n)\) samples are enough and necessary to learn an arbitrary distribution; but who would have thought that \(\Theta(n/\log n)\) was the right answer to anything? [7]). And game changers (general theorems that apply to many questions or blackbox lemmas are really nice to have in one’s toolbox).
And that’s barely the tip of the iceberg! For more in-depth or better written prose, the interested Internet wanderer may want to consult one of the following (usual non-exhaustivity caveats apply):
- Ronitt Rubinfeld’s introduction [1]
- Dana Ron’s survey on Property testing [2] (Section 11.4)
- Oded Goldreich’s upcoming book [3] (Chapter 11)
- or my own survey [4] on distribution testing (but I cannot promise better written prose for this one).
For the more video-inclined, there are also quite a few survey talks available online, the most recent I know of being this talk by Ronitt Rubinfeld at COLT’16.
A Brief Comparison
Note: this section is taken verbatim from my survey [4] (Section 1.2).
It is natural to wonder how the above approach to distribution testing compares to classic methods and formulations, as studied in Statistics. While the following will not be a thorough comparison, it may help shed some light on the difference.
- Null and alternative hypotheses. The standard take on hypothesis testing, simple hypothesis testing, relies on defining two classes of distributions, the null and alternative hypotheses \(\mathcal{H}_0\) and \(\mathcal{H}_1\). A test statistic is then tailored specifically to these two classes, in order to optimally distinguish between \(\mathcal{H}_0\) and \(\mathcal{H}_1\)— that is, under the underlying assumption that the unknown distribution \(D\in\mathcal{H}_0\cup\mathcal{H}_1\).
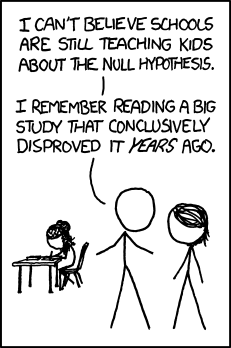
Something like that.
The test then rejects the null hypothesis \(\mathcal{H}_0\) if statistical evidence is obtained that \(D\notin\mathcal{H}_0\). In this view, the distribution testing formulation would be to set \(\mathcal{H}_0\) to be the property \(\mathcal{P}\) to be tested, and define the alternative hypothesis as “everything far from \(\mathcal{P}\).” In this sense, the latter captures a much more adversarial setting, where almost no structure is assumed on the alternative hypothesis— setting known in Statistics as composite hypothesis testing.
- Small-sample regime. A second and fundamental difference resides in the emphasis given to the question. Traditionally, statisticians tend to focus on asymptotic analysis, characterizing— often exactly— the rate of convergence of the statistical tests under the alternative hypothesis, as the number of samples \(m\) grows to infinity. Specifically, the goal is to pinpoint the error exponent \(\rho\) such that the probability of error (failing to reject the null hypothesis) asymptotically decays as \(e^{-\rho m}\). However, this asymptotic behavior will generally only hold for values of \(m\) greater than the size of the domain (“alphabet”). In contrast, the computer science focus is on the small-sample regime, where the number of samples available is small with regard to the domain size, and one aims at achieving a fixed probability of error.
- Algorithmic flavor. At a more practical level, a third point on which the two approaches deviate is the set of techniques used in order to tackle the question. Namely, the Statistics literature very often relies on relatively simple-looking and “natural” tests and estimators, which need not be computationally efficient. (This is for instance the case for the generalized likelihood ratio test that requires to compute the maximum likelihood of the sequence of samples obtained under the two hypotheses \(\mathcal{H}_0\) and \(\mathcal{H}_1\); which is not in general tractable.) On the other hand, works in distribution testing are predominantly algorithmic, with a computational emphasis on the testing algorithms thus obtained.
Exciting times!
And while the above has hinted at all that has been done so far in the area, there is still plenty ahead — many new questions to be posed, answered, revisited under another light, tackled with new insights and techniques, and connections to be made. As a small sample (sic), we list below a few of the recent developments or trends that herald these exciting times.
New tools
- Appeared in the 1800’s thanks to Pearson, testers based on (some tailored variant of) a \(\chi^2\)-based statistic are now aplenty. Yielding simple, concise, very often optimal testing algorithms… [8], [9], [10], [11]…
- Information theory. Techniques and results from this related area have started to permeate distribution testing— mostly to prove lower bounds in an easier or conceptually simpler way. [12], [13,14]
- They were there from the beginning. Now, the \(\ell_2\)-testing subroutines strike back. (For neat, elegant testing algorithms that use \(\ell_2\) as a proxy “in the right way.”) [5,6,15], [14]
New models
What if modeling the access to the data as a source of i.i.d. samples was not enough? Because we can— the situation allows it— or because we want— what we are trying to achieve is best modeled a bit differently? If we get more ways to query it, can we do more, and by how much?
This recent line of work [20,21,22,23,…] offers many challenges, ranging from the right way to ask (what to model, and how?) to the right way to answer (intuition if often off the hook, in these uncharted waters?). New tools to design, intuition to build, and problems to consider…
New questions
And of course, even if many of the “classic” problems have by now been fully understood, there are many more that await… from class testing [16,11,17,18] to twists on old questions (uneven sample size for closeness?) to new questions entirely [19], there is no shortage. Not to forget there is a stronger connection with practice to build, and asking the “real-worlders” what makes sense to consider is also unlikely to stop producing challenges.
[1] Ronitt Rubinfeld. Taming Big Probability Distributions. XRDS, 19(1):24-28, 2012. http://xrds.acm.org/article.cfm?aid=2331052
[2] Dana Ron. Algorithmic and Analysis Techniques in Property Testing. Foundations and Trends in Theoretical Computer Science: Vol. 5: No. 2, pp 73-205. 2010. http://dx.doi.org/10.1561/0400000029
[3] Oded Goldreich. Introduction to Property Testing. (In preparation) http://www.wisdom.weizmann.ac.il/~oded/pt-intro.html
[4] Clément Canonne. A Survey on Distribution Testing: Your Data is Big. But is it Blue? 2015. http://eccc.hpi-web.de/report/2015/063/
[5] Oded Goldreich and Dana Ron. On testing expansion in bounded-degree graphs. Electronic Colloquium on Computational Complexity (ECCC), 7:20, 2000.
[6] Tuğkan Batu, Lance Fortnow, Ronitt Rubinfeld, Warren D. Smith, and Patrick White. Testing that distributions are close. In Proceedings of FOCS, pages 189-197, 2000.
[7] Gregory Valiant and Paul Valiant. The power of linear estimators. In Proceedings of FOCS, pages 403-412, October 2011.
[8] Siu-On Chan, Ilias Diakonikolas, Gregory Valiant, and Paul Valiant. Optimal algorithms for testing closeness of discrete distributions. In Proceedings of SODA, pages 1193-1203. Society for Industrial and Applied Mathematics (SIAM), 2014.
[9] Gregory Valiant and Paul Valiant. An automatic inequality prover and instance optimal identity testing. In Proceedings of FOCS, 2014.
[10] Ilias Diakonikolas, Daniel M. Kane, and Vladimir Nikishkin. Testing Identity of Structured Distributions. In Proceedings of SODA, pages 1841-1854. Society for Industrial and Applied Mathematics (SIAM), 2015.
[11] Jayadev Acharya, Constantinos Daskalakis, Gautam Kamath. Optimal Testing for Properties of Distributions. In Advances in Neural Information Processing Systems (NIPS), 2015.
[12] Yihong Wu and Pengkun Yang. Minimax rates of entropy estimation on large alphabets via best polynomial approximation. ArXiV, abs/1407.0381, 2014.
[13] Yihong Wu and Pengkun Yang. Chebyshev polynomials, moment matching, and optimal estimation of the unseen. ArXiV, abs/1504.01227, 2015.
[14] Ilias Diakonikolas and Daniel Kane. A New Approach for Testing Properties of Discrete Distributions. In Proceedings of FOCS, 2016. (To appear. Also available as abs/1601.05557)
[15] Tuğkan Batu, Eldar Fischer, Lance Fortnow, Ravi Kumar, Ronitt Rubinfeld, and Patrick White. Testing random variables for independence and identity. InProceedings of FOCS, pages 442-451, 2001.
[16] Tuğkan Batu, Ravi Kumar, and Ronitt Rubinfeld. Sublinear algorithms for testing monotone and unimodal distributions. In Proceedings of STOC, pages 381-390, New York, NY, USA, 2004. ACM.
[17] Clément L. Canonne, Ilias Diakonikolas, Themis Gouleakis, and Ronitt Rubinfeld. Testing Shape Restrictions of Discrete Distributions, In Proceedings of STACS, pages 25:1–25:14, 2016.
[18] MIT Theory Blog. Distribution Testing: Do It With Class! https://mittheory.wordpress.com/2015/08/09/distribution-testing-do-it-with-class/
[19] Maryam Aliakbarpour, Eric Blais, Ronitt Rubinfeld. Learning and Testing Junta Distributions. In Proceedings of COLT, 2016. pp. 19–46, 2016
[20] Reut Levi, Dana Ron, and Ronitt Rubinfeld. Testing properties of collections of distributions. Theory of Computing, 9:295-347, 2013.
[21] Sourav Chakraborty, Eldar Fischer, Yonatan Goldhirsh, and Arie Matsliah. On the power of conditional samples in distribution testing. In Proceedings of ITCS, pages 561-580, New York, NY, USA, 2013. ACM.
[22] Clément L. Canonne, Dana Ron, and Rocco A. Servedio. Testing probability distributions using conditional samples. SIAM Journal on Computing, 2015.
[23] Clément L. Canonne and Ronitt Rubinfeld. Testing probability distributions underlying aggregated data. In Proceedings of ICALP, pages 283-295, 2014.